Understanding Model Capability Extraction in AI-Powered IP Portfolio Management Software

With the advent of AI patent search technology such as patlytics.ai, IP portfolio management software has significantly improved, offering organizations deeper insights into their patents and trademarks. Central to these advancements is a process known as model capability extraction. The process was detailed by Microsoft in a patent published in April 2024, and filed in October 2022.
What is Model Capability Extraction?
Model capability extraction involves identifying and analyzing the capabilities of a machine learning model to understand its strengths and limitations. As described in the abstract of the patent, model capability extraction is “the indirect querying of models to determine capabilities possessed by the model”. The key aspects of model capability extraction include:
- Performance Evaluation
- Explainability and Interpretability
- Bias Detection
- Behavior Analysis
- Capability Mapping
Applying Model Capability Extraction to IP Portfolio Management Software
- Performance Evaluation in Patent Classification and Analysis:
- AI models are extensively used to classify patents based on their technical domains. Through capability extraction, we can measure their classification accuracy and identify improvement opportunities.
- For example, models trained to identify relevant prior art can be evaluated against standardized benchmarks to ensure that the software supports patent examiners or legal teams appropriately.
- This performance evaluation is crucial in a landscape where the accuracy and speed of patent processing directly impact innovation cycles and market entry times. By systematically evaluating AI models, organizations can pinpoint performance bottlenecks and refine their models to increase throughput and reduce errors, thereby streamlining the patent examination process.
- Explainability for Strategic Decision-Making:
- By using feature importance analysis, organizations can gain insights into the factors driving a model’s recommendations, such as identifying high-value patents for licensing.
- This transparency is vital for making informed strategic decisions that align with business goals. For instance, understanding why a model prioritizes certain patents for litigation support or licensing can help firms tailor their legal strategies to protect or capitalize on specific intellectual properties.
- Moreover, explainability builds trust among stakeholders, who can see and understand the rationale behind automated decisions, reducing conflicts and promoting smoother operations.
- Bias Detection in Patent Valuation:
- AI models may inadvertently inherit biases present in historical patent data, potentially affecting valuation predictions.
- Model capability extraction enables businesses to detect and correct biases, ensuring fair valuation across different technological domains and geographical regions.
- Addressing biases is not only a matter of fairness but also a strategic imperative. For example, identifying and correcting geographical bias ensures that innovations from emerging markets are valued appropriately, helping companies recognize and seize global opportunities. By systematically addressing these biases, firms can ensure that their valuation algorithms are robust, fair, and aligned with global standards.
- Behavior Analysis in Trademark Monitoring:
- Trademark infringement detection requires models that can recognize slight variations in logos or names.
- Through capability extraction, organizations can identify adversarial vulnerabilities or scenarios where the model struggles, thereby refining the model to improve detection accuracy.
- This analysis is crucial in protecting brand identity and intellectual property in a global marketplace where infringement tactics are constantly evolving. By understanding the specific weaknesses of their models, companies can implement targeted improvements, such as training on more diverse datasets or tweaking image recognition algorithms to detect subtle misrepresentations more effectively.
- Capability Mapping for Efficient Portfolio Management:
- By mapping the strengths of different AI models, businesses can allocate tasks strategically. For instance, models particularly adept at prior art searches can be leveraged to accelerate freedom-to-operate (FTO) analysis.
- This mapping ensures that each AI model within the IP portfolio management software is utilized to its fullest potential.
- Capability mapping allows firms to optimize their resource allocation, focusing on leveraging each model’s strengths in various aspects of IP management. This strategic approach not only improves efficiency but also enhances the overall effectiveness of IP portfolio management, helping firms maintain a competitive edge in their respective industries.
Conclusion
Model capability extraction is a vital process that helps organizations harness the full potential of AI models embedded in IP portfolio management software. By understanding the strengths and limitations of these models, businesses can ensure more accurate patent classification, strategic decision-making, and unbiased valuation, ultimately leading to a stronger and more efficiently managed IP portfolio.
Deliver winning IP outcomes
The Premier AI-Powered
Patent Platform
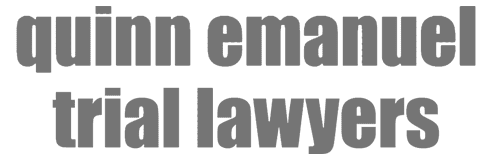

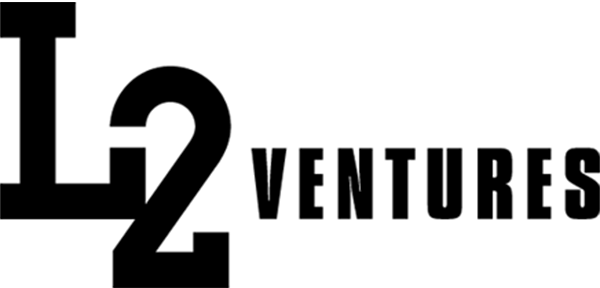
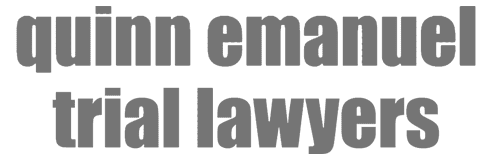

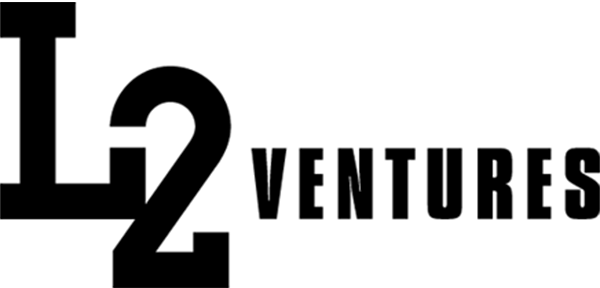
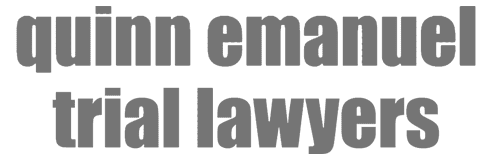

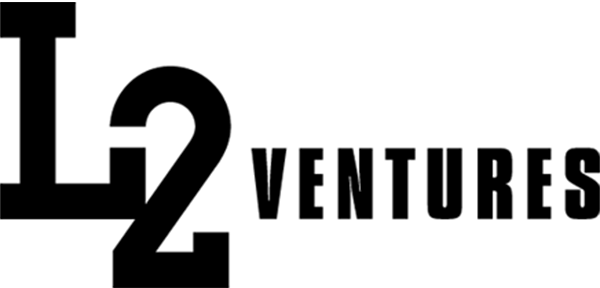
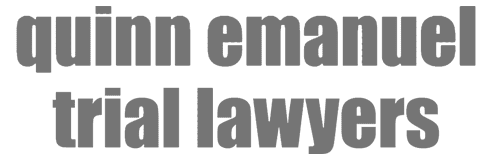

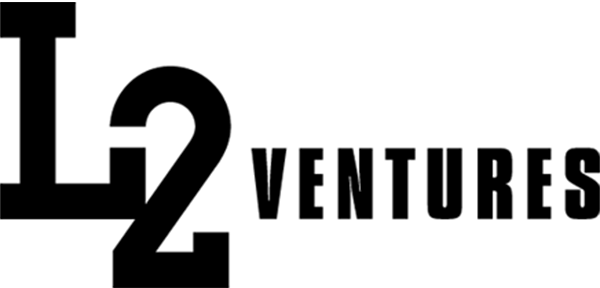